 Книжкові видання та компакт-диски  Журнали та продовжувані видання  Автореферати дисертацій  Реферативна база даних  Наукова періодика України  Тематичний навігатор  Авторитетний файл імен осіб
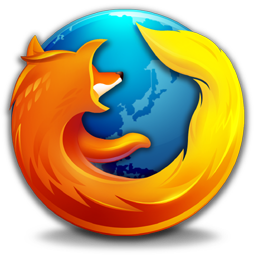 |
Для швидкої роботи та реалізації всіх функціональних можливостей пошукової системи використовуйте браузер "Mozilla Firefox" |
|
|
Повнотекстовий пошук
Пошуковий запит: (<.>A=Protsenko M$<.>) |
Загальна кількість знайдених документів : 10
Представлено документи з 1 до 10
|
1. |
Protsenko M. B. Investigation of directional properties of curved antenna arrays [Електронний ресурс] / M. B. Protsenko, А. А. Iaremenko // Вимірювальна та обчислювальна техніка в технологічних процесах. - 2014. - № 2. - С. 99-102. - Режим доступу: http://nbuv.gov.ua/UJRN/vott_2014_2_16
| 2. |
Protsenko M. The Pavlovs merchants: From the history of hotel business in Kharkiv [Електронний ресурс] / M. Protsenko // Вісник Харківського національного університету імені В. Н. Каразіна. Серія : Історія. - 2014. - № 1134, вип. 49(спец. вип.). - С. 230-237. - Режим доступу: http://nbuv.gov.ua/UJRN/VKhIS_2014_1134_49(spets
| 3. |
Protsenko M. B. Analysis of equivalent aperture of curvilinear antenna arrays [Електронний ресурс] / M. B. Protsenko, А. A. Iaremenko // Наукові праці ОНАЗ ім. О. С. Попова. - 2014. - № 2. - С. 27-35. - Режим доступу: http://nbuv.gov.ua/UJRN/Nponaz_2014_2_5
| 4. |
Sharapov S. Improvement of the Vacuum Cooling System for Biodiesel Production [Електронний ресурс] / S. Sharapov, M. Starchenko, M. Protsenko, V. Panchenko, V. Kovtun // Journal of engineering sciences. - 2019. - Vol. 6, Iss. 1. - С. F6-F11. - Режим доступу: http://nbuv.gov.ua/UJRN/jes_2019_6_1_18 Вирішено актуальну проблему підвищення ефективності вакуумних систем охолодження установок виробництва біодизельного палива шляхом застосування вакуумних агрегатів на базі рідинно-парового ежектора, що працює за принципом струминної термокомпресії. Мета роботи - підтвердження доцільності застосування вакуумних агрегатів на базі рідинно-парового ежектора, що працює за принципом струминної термокомпресії, у вакуумних системах охолодження установок виробництва біодизельного палива. Надано опис базової схеми системи охолодження установки виробництва біодизельного палива, яка містить трьохступеневий пароструминний ежектор. Запропоновано схему на базі рідинно-парового ежектора. Виконано порівняльний аналіз вакуумних систем охолодження установок виробництва біодизельного палива, схеми яких порівнювались, у тому числі на базі існуючих триступеневих пароструминних ежекторів і нового одноступеневого вакуумного агрегату на базі рідинно-парового ежектора. Як порівняльний аналіз застосовано ексергетичний метод оцінювання ефективності застосування запропонованого устаткування, оскільки у робочому процесі відбувається перетворення двох видів енергії: електричної (для приводу насоса) і теплової (для підігріву робочої рідини активного потоку у теплообміннику-підігрівачі). У результаті досягнуті більші показники ефективності застосування нової технології за оптимізаційним параметром - проміжним тиском між рідинно-паровим ежектором та рідинно-кільцевим вакуумним насосом.
| 5. |
Vanyeyev S. M. Data Measuring System for Torque Measurement on Running Shafts Based on a Non-Contact Torsional Dynamometer [Електронний ресурс] / S. M. Vanyeyev, D. V. Miroshnichenko, T. S. Rodymchenko, M. Protsenko, D. V. Smolenko // Journal of engineering sciences. - 2019. - Vol. 6, Iss. 2. - С. E17-E23. - Режим доступу: http://nbuv.gov.ua/UJRN/jes_2019_6_2_13 Необхідність вимірювання потужності, переданої валом, що обертається, призвела до важливості застосовування пристроїв для вимірювання обертального моменту на валу. При цьому, особливого значення набуває вимірювання потужності на високошвидкісних установках, де у більшості випадків традиційні системи вимірювання або непридатні, або мають недостатню точність. У даний час при дослідженнях турбомашин широко розповсюджені інформаційно-вимірювальні системи. Вони дозволяють отримувати, обробляти, передавати, запам'ятовувати і відображати вимірювальну інформацію. Їх застосування актуальне у зв'язку із пріоритетністю експериментального вивчення і подальшого моделювання характеристик та показників ефективності розширювальних машин. Метою даної роботи є розроблення і створення інформаційно-вимірювальної системи для вимірювання обертального моменту на валу розширювальних машин, що обертається з великою швидкістю, за допомогою безконтактного торсіонного динамометра (тензометричної муфти). Наведені результати розроблення інформаційно-вимірювальної системи, виконаний теоретичний аналіз і результати практичного застосування безконтактного тензометричного динамометра, призначеного для вимірювання обертального моменту на валу розширювальних машин малої потужності за умов стендових випробувань. Додатково розглянуто питання проектування, тарування і застосування спроектованого динамометра.
| 6. |
Slyusar V. Improvement of the object recognition model on aerophotos using deep convolutional neural network [Електронний ресурс] / V. Slyusar, M. Protsenko, A. Chernukha, P. Kovalov, P. Borodych, S. Shevchenko, O. Chernikov, S. Vazhynskyi, O. Bogatov, K. Khrustalev // Eastern-european journal of enterprise technologies. - 2021. - № 5(2). - С. 6-21. - Режим доступу: http://nbuv.gov.ua/UJRN/Vejpte_2021_5(2)__3 Detection and recognition of objects in images is the main problem to be solved by computer vision systems. As part of solving this problem, the model of object recognition in aerial photographs taken from unmanned aerial vehicles has been improved. A study of object recognition in aerial photographs using deep convolutional neural networks has been carried out. Analysis of possible implementations showed that the AlexNet 2012 model (Canada) trained on the ImageNet image set (China) is most suitable for this problem solution. This model was used as a basic one. The object recognition error for this model with the use of the ImageNet test set of images amounted to 15 %. To solve the problem of improving the effectiveness of object recognition in aerial photographs for 10 classes of images, the final fully connected layer was modified by rejection from 1,000 to 10 neurons and additional two-stage training of the resulting model. Additional training was carried out with a set of images prepared from aerial photographs at stage 1 and with a set of VisDrone 2021 (China) images at stage 2. Optimal training parameters were selected: speed (step) (0,0001), number of epochs (100). As a result, a new model under the proposed name of AlexVisDrone was obtained. The effectiveness of the proposed model was checked with a test set of 100 images for each class (the total number of classes was 10). Accuracy and sensitivity were chosen as the main indicators of the model effectiveness. As a result, an increase in recognition accuracy from 7 % (for images from aerial photographs) to 9 % (for the VisDrone 2021 set) was obtained which has indicated that the choice of neural network architecture and training parameters was correct. The use of the proposed model makes it possible to automate the process of object recognition in aerial photographs. In the future, it is advisable to use this model at ground stations of unmanned aerial vehicle complex control when processing aerial photographs taken from unmanned aerial vehicles, in robotic systems, in video surveillance complexes and when designing unmanned vehicle systems.
| 7. |
Slyusar V. Construction of an advanced method for recognizing monitored objects by a convolutional neural network using a discrete wavelet transform [Електронний ресурс] / V. Slyusar, M. Protsenko, A. Chernukha, S. Gornostal, S. Rudakov, S. Shevchenko, O. Chernikov, N. Kolpachenko, V. Timofeyev, R. Artiukh // Eastern-european journal of enterprise technologies. - 2021. - № 4(9). - С. 65-77. - Режим доступу: http://nbuv.gov.ua/UJRN/Vejpte_2021_4(9)__9 The tasks that unmanned aircraft systems solve include the detection of objects and determining their state. This paper reports an analysis of image recognition methods in order to automate the specified process. Based on the analysis, an improved method for recognizing images of monitored objects by a convolutional neural network using a discrete wavelet transform has been devised. Underlying the method is the task of automating image processing in unmanned aircraft systems. The operability of the proposed method was tested using an example of processing an image (aircraft, tanks, helicopters) acquired by the optical system of an unmanned aerial vehicle. A discrete wavelet transform has been used to build a database of objects' wavelet images and train a convolutional neural network based on them. That has made it possible to improve the efficiency of recognition of monitored objects and automate a given process. The effectiveness of the improved method is achieved by preliminary decomposition and approximation of the digital image of the monitored object by a discrete wavelet transform. The stages of a given method include the construction of a database of the wavelet images of images and training a convolutional neural network. The effectiveness of recognizing the monitored objects' images by the improved method was tested on a convolutional neural network, which was trained with images of 300 monitored objects. In this case, the time to make a decision, based on the proposed method, decreased on average from 0,7 to 0,84 s compared with the artificial neural networks ResNet and ConvNets. The method could be used in the information processing systems in unmanned aerial vehicles that monitor objects; in robotic complexes for various purposes; in the video surveillance systems of important objects.
| 8. |
Slyusar V. Improving a neural network model for semantic segmentation of images of monitored objects in aerial photographs [Електронний ресурс] / V. Slyusar, M. Protsenko, A. Chernukha, V. Melkin, O. Petrova, M. Kravtsov, S. Velma, N. Kosenko, O. Sydorenko, M. Sobol // Eastern-european journal of enterprise technologies. - 2021. - № 6(2). - С. 86-95. - Режим доступу: http://nbuv.gov.ua/UJRN/Vejpte_2021_6(2)__9 This paper considers a model of the neural network for semantically segmenting the images of monitored objects on aerial photographs. Unmanned aerial vehicles monitor objects by analyzing (processing) aerial photographs and video streams. The results of aerial photography are processed by the operator in a manual mode; however, there are objective difficulties associated with the operator's handling a large number of aerial photographs, which is why it is advisable to automate this process. Analysis of the models showed that to perform the task of semantic segmentation of images of monitored objects on aerial photographs, the U-Net model (Germany), which is a convolutional neural network, is most suitable as a basic model. This model has been improved by using a wavelet layer and the optimal values of the model training parameters: speed (step) - 0,001, the number of epochs - 60, the optimization algorithm - Adam. The training was conducted by a set of segmented images acquired from aerial photographs (with a resolution of 6,000 x 4,000 pixels) by the Image Labeler software in the mathematical programming environment MATLAB R2020b (USA). As a result, a new model for semantically segmenting the images of monitored objects on aerial photographs with the proposed name U-NetWavelet was built. The effectiveness of the improved model was investigated using an example of processing 80 aerial photographs. The accuracy, sensitivity, and segmentation error were selected as the main indicators of the model's efficiency. The use of a modified wavelet layer has made it possible to adapt the size of an aerial photograph to the parameters of the input layer of the neural network, to improve the efficiency of image segmentation in aerial photographs; the application of a convolutional neural network has allowed this process to be automatic.
| 9. |
Slyusar V. Improving the model of object detection on aerial photographs and video in unmanned aerial systems [Електронний ресурс] / V. Slyusar, M. Protsenko, A. Chernukha, V. Melkin, O. Biloborodov, M. Samoilenko, O. Kravchenko, H. Kalynychenko, A. Rohovyi, M. Soloshchuk // Eastern-European journal of enterprise technologies. - 2022. - № 1(9). - С. 24–34. - Режим доступу: http://nbuv.gov.ua/UJRN/Vejpte_2022_1(9)__5 This paper considers a model of object detection on aerial photographs and video using a neural network in unmanned aerial systems. The development of artificial intelligence and computer vision systems for unmanned systems (drones, robots) requires the improvement of models for detecting and recognizing objects in images and video streams. The results of video and aerial photography in unmanned aircraft systems are processed by the operator manually but there are objective difficulties associated with the operator's processing of a large number of videos and aerial photographs, so it is advisable to automate this process. Analysis of neural network models has revealed that the YOLOv5x model (USA) is most suitable, as a basic model, for performing the task of object detection on aerial photographs and video. The Microsoft COCO suite (USA) is used to train this model. This set contains more than 200,000 images across 80 categories. To improve the YOLOv5x model, the neural network was trained with a set of VisDrone 2021 images (China) with the choice of such optimal training parameters as the optimization algorithm SGD; the initial learning rate (step) of 0,0005; the number of epochs of 25. As a result, a new model of object detection on aerial photographs and videos with the proposed name VisDroneYOLOv5x was obtained. The effectiveness of the improved model was studied using aerial photographs and videos from the VisDrone 2021 set. To assess the effectiveness of the model, the following indicators were chosen as the main indicators: accuracy, sensitivity, the estimation of average accuracy. Using a convolutional neural network has made it possible to automate the process of object detection on aerial photographs and video in unmanned aerial systems.
| 10. |
Borshchov V. M. Nanopolymer optically transparent structures, systems and devices [Електронний ресурс] / V. M. Borshchov, O. M. Listratenko, M. A. Protsenko, I. T. Tymchuk, O. V. Kravchenko, O. V. Suddia, I. V. Borshchov, M. I. Slipchenko // Radiotekhnika. - 2023. - Iss. 213. - С. 56-64. - Режим доступу: http://nbuv.gov.ua/UJRN/rvmnts_2023_213_8
|
|
|